Note
Click here to download the full example code
A quick example¶
Amputation is the opposite of imputation: the generation of missing values in complete datasets. That is useful in an experimental setting where you want to evaluate the effect of missing values on the outcome of a model.
MultivariateAmputation
is designed following scikit-learn’s fit
and transform
paradigm, and can therefore seamless be integrated in a larger data processing pipeline.
Here, we give a short demonstration. A more extensive example can be found in this example. For people who are familiar with the implementation of multivariate amputation in R-function ampute, this blogpost gives an overview of the similarities and differences with MultivariateAmputation
. Inspection of an incomplete dataset can be done with mdPatterns
.
Note that the amputation methodology itself is proposed in Generating missing values for simulation purposes and in The dance of the mechanisms.
# Author: Rianne Schouten <https://rianneschouten.github.io/>
# Co-Author: Davina Zamanzadeh <https://davinaz.me/>
Transforming one dataset¶
Multivariate amputation of one dataset can directly be performed with
fit_transform
. Inspection of an incomplete dataset can be done withmdPatterns
. By default,MultivariateAmputation
generates 1 pattern with MAR missingness in 50% of the data rows for 50% of the variables.
import numpy as np
from pyampute.ampute import MultivariateAmputation
from pyampute.exploration.md_patterns import mdPatterns
seed = 2022
rng = np.random.default_rng(seed)
m = 1000
n = 10
X_compl = rng.standard_normal((m, n))
ma = MultivariateAmputation(seed=seed)
X_incompl = ma.fit_transform(X_compl)
mdp = mdPatterns()
patterns = mdp.get_patterns(X_incompl)
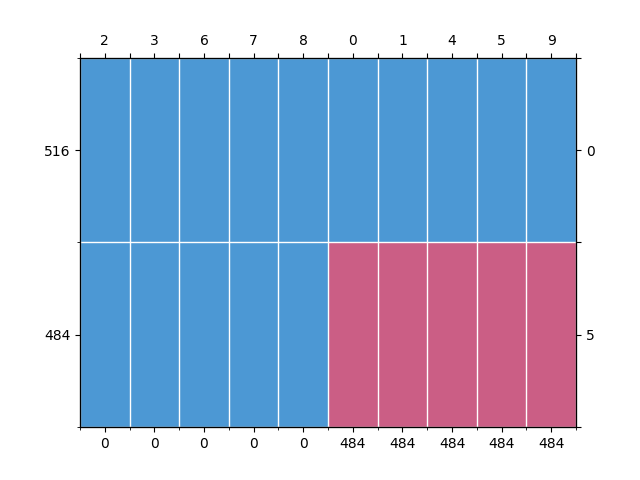
Out:
/home/dav/research/pyampute/pyampute/exploration/md_patterns.py:120: FutureWarning: The frame.append method is deprecated and will be removed from pandas in a future version. Use pandas.concat instead.
group_values = group_values.append(colsums, ignore_index=True)
A separate fit and transform¶
Integration in a larger pipeline requires separate
fit
andtransform
functionality.
from sklearn.model_selection import train_test_split
X_compl_train, X_compl_test = train_test_split(X_compl, random_state=2022)
ma = MultivariateAmputation()
ma.fit(X_compl_train)
X_incompl_test = ma.transform(X_compl_test)
Integration in a pipeline¶
A short pipeline may look as follows.
from sklearn.pipeline import make_pipeline
from sklearn.impute import SimpleImputer
import matplotlib.pyplot as plt
pipe = make_pipeline(MultivariateAmputation(), SimpleImputer())
pipe.fit(X_compl_train)
X_imp_test = pipe.transform(X_compl_test)
By default, SimpleImputer
imputes with the mean of the observed data. It is therefore like that we find the median in 50% of the rows (of the test set, which contains 25% of m) for 50% of the variables.
medians = np.nanmedian(X_imp_test, axis=0)
print(np.sum(X_imp_test == medians[None, :], axis=0))
Out:
[ 0 0 120 120 120 0 120 120 0 0]
For more information about pyampute
’s parameters, see A mapping from R-function ampute to pyampute. To learn how to design a more thorough experiment, see Evaluating missing values with grid search and a pipeline.
Total running time of the script: ( 0 minutes 0.226 seconds)